This plan includes
- Limited free courses access
- Play & Pause Course Videos
- Video Recorded Lectures
- Learn on Mobile/PC/Tablet
- Quizzes and Real Projects
- Lifetime Course Certificate
- Email & Chat Support
What you'll learn?
- How algorithms influence your life and decisions.
- How software algorithm deployed by organizations can be audited to find errors, misuse or bias
- Frames in which editorial transparency can be achieved
Course Overview
Platform power, algorithm accountability and reporting, for Non-Technicals, Leaders, Managers, Freshers and Beginners.
In the course before this one, you have learnt so much about what algorithms are and how they work. In this course we will be discussing the algorithmic power behind many social media applications and so much more;
? How algorithms influence your life and decisions.
? How to call these algorithms to question.
? How to earn a living by auditing or questioning how and why these social media algorithms behave the way they do, or make decisions for the society that could be dangerous down the line.
This course is a single installation of a broader advanced 3-part course called ‘Automated Content Production and News Algorithms’. It consists of 4 modules and 20 learning videos including the transcript and practice questions.
This Part of the course covers;
The algorithmic power behind Tiktok
The algorithmic power behind Twitter
The algorithmic power behind Triller
The algorithmic power behind Youtube
The algorithmic power behind Facebook.
Algorithmic accountability reporting methods
Techniques to critique, investigate and report an algorithm
Understanding how to validate and document the occurrence of errors in technology or software applications
Editorial responsibility and algorithmic transparency
Frames in which editorial transparency can be achieved
How software algorithm deployed by organizations can be audited to find errors, misuse or bias
Case Study 1: How minority neighborhoods pay higher car insurance premiums than the privileged with the same risk factor
Case Study 2: A software meant to predict future criminals is biased against blacks
Analysis of the algorithm used by prisons to create risk scores
Angles for investigating algorithms
You will get 20 videos of learning content with transcript and practice questions
Besides the video materials, there are also transcripts of the videos, and practice questions to help guide and reinforce your learning.
Buy this course already and get started.
Pre-requisites
- Willingness to learn and practice learning
Target Audience
- Non-Technicals
- Leaders
- Managers
- Freshers
Curriculum 25 Lectures 00:19:54
Section 1 : Platform power
- Lecture 2 :
- Triller
- Lecture 3 :
- Lecture 4 :
- Lecture 5 :
- YouTube
- Lecture 6 :
- TikTok
- Lecture 7 :
- Quiz
Section 2 : Algorithm Accountability
- Lecture 1 :
- Algorithmic Accountability Reporting Methods
- Lecture 2 :
- Algorithmic Accountability Reporting Methods- Overview
- Lecture 3 :
- Techniques to Critique and Investigting an Algorithm
- Lecture 4 :
- Quiz
- Lecture 5 :
- Techniques to Critique and Investigting an Algorithm
Section 3 : Editorial Responsibility and Algorithmic Transparency
- Lecture 1 :
- Editorial Responsibility and Algorithmic Transparency
- Lecture 2 :
- Editorial Responsibility and Algorithmic Transparency- An Overview
- Lecture 3 :
- How Publishers are Taking Responsibility
- Lecture 4 :
- Frames in which transparency can be achieved
- Lecture 5 :
- Quiz
Section 4 : Algorithm Beats
- Lecture 1 :
- Introduction
- Lecture 2 :
- An Overview of Algorithm Beats
- Lecture 3 :
- Algorithm Beats Case 1
- Lecture 4 :
- Algorithm Beats Case 2
- Lecture 5 :
- Algorithm Beats Case 3
- Lecture 6 :
- Angles for Investigating Algorithms
- Lecture 7 :
- Quiz
Our learners work at
Frequently Asked Questions
How do i access the course after purchase?
It's simple. When you sign up, you'll immediately have unlimited viewing of thousands of expert courses, paths to guide your learning, tools to measure your skills and hands-on resources like exercise files. There’s no limit on what you can learn and you can cancel at any time.Are these video based online self-learning courses?
Yes. All of the courses comes with online video based lectures created by certified instructors. Instructors have crafted these courses with a blend of high quality interactive videos, lectures, quizzes & real world projects to give you an indepth knowledge about the topic.Can i play & pause the course as per my convenience?
Yes absolutely & thats one of the advantage of self-paced courses. You can anytime pause or resume the course & come back & forth from one lecture to another lecture, play the videos mulitple times & so on.How do i contact the instructor for any doubts or questions?
Most of these courses have general questions & answers already covered within the course lectures. However, if you need any further help from the instructor, you can use the inbuilt Chat with Instructor option to send a message to an instructor & they will reply you within 24 hours. You can ask as many questions as you want.Do i need a pc to access the course or can i do it on mobile & tablet as well?
Brilliant question? Isn't it? You can access the courses on any device like PC, Mobile, Tablet & even on a smart tv. For mobile & a tablet you can download the Learnfly android or an iOS app. If mobile app is not available in your country, you can access the course directly by visting our website, its fully mobile friendly.Do i get any certificate for the courses?
Yes. Once you complete any course on our platform along with provided assessments by the instructor, you will be eligble to get certificate of course completion.For how long can i access my course on the platform?
You require an active subscription to access courses on our platform. If your subscription is active, you can access any course on our platform with no restrictions.Is there any free trial?
Currently, we do not offer any free trial.Can i cancel anytime?
Yes, you can cancel your subscription at any time. Your subscription will auto-renew until you cancel, but why would you want to?
Instructor
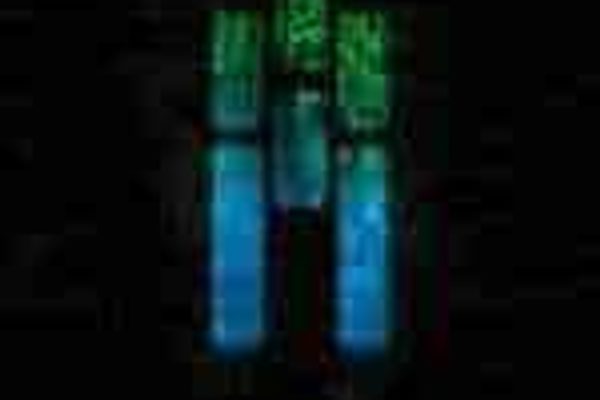
97255 Course Views
3 Courses